18 min read
The Ultimate Guide to Data Mesh
Written by: Micah Horner, Product Marketing Manager, TimeXtender - December 15, 2023
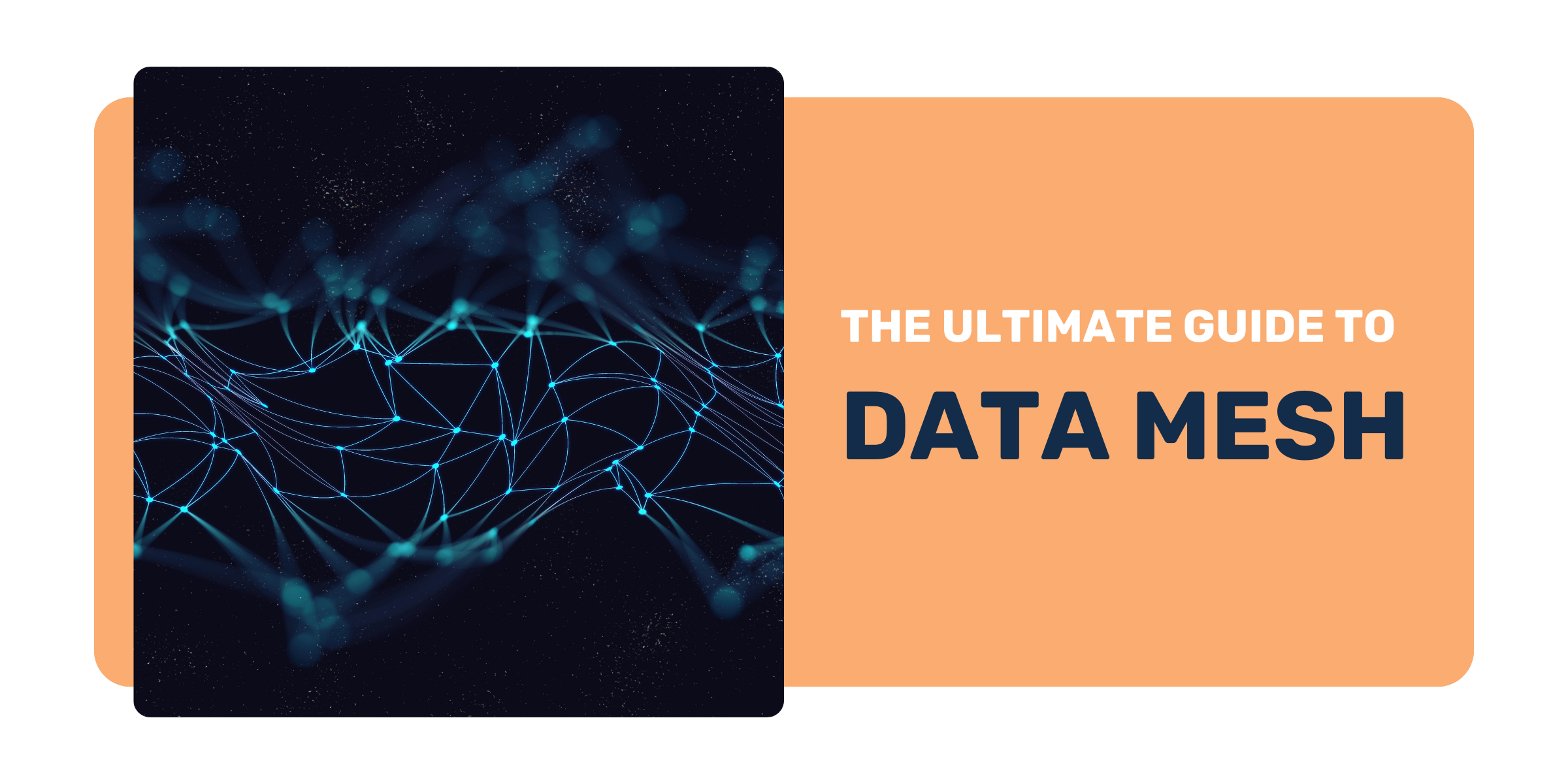
The rising popularity of Data Mesh in the world of data architecture and management signifies a paradigm shift in how data is handled within organizations. This novel approach, centered around decentralizing control and ownership of data, is rapidly gaining traction for its ability to address the limitations and challenges faced by traditional centralized data systems. Unlike centralized models that often lead to bottlenecks, a lack of agility, and scaling challenges, Data Mesh offers a refreshing alternative.
Data Mesh is not a technological solution; it represents a set of organizational principles rooted in "domain-driven design". This approach reimagines data ownership, treating it as a product with domain-specific control and accountability. Such a transformation in data management philosophy is appealing to organizations looking to overcome the inefficiencies of centralized systems and seeking more agile, responsive, and scalable data operations.
The increasing adoption of Data Mesh reflects a broader move towards systems that empower domain experts, promote decentralized decision-making, and ensure data is managed more effectively and contextually. As organizations continue to grapple with the complexities of big data and the need for more dynamic data infrastructures, Data Mesh stands out as a compelling approach, redefining the future of data management.
What Is Data Mesh?
Data Mesh represents a significant shift in the field of data architecture and management. It is a novel approach focused on decentralizing the control and ownership of data across an organization.
Unlike traditional centralized systems, Data Mesh treats data as a product, emphasizing domain-specific control and accountability. This approach aims to overcome the challenges faced by centralized data systems, such as bottlenecks, lack of agility, and challenges in scaling data operations.
It is important to understand that Data Mesh is neither a specific technology nor an architectural framework; rather, it is a set of organizational principles rooted in "domain-driven design."
Key Principles of Data Mesh
The Data Mesh model transforms how organizations approach data management by embodying several key principles, each designed to optimize data utility and efficiency:
1. Decentralized Data Ownership
Data ownership in Data Mesh is distributed among various domains within an organization. Each domain, such as sales, marketing, finance, or operations, acts as a custodian of its own data. This means the domain is responsible for creating, maintaining, and managing its data assets.
By decentralizing data ownership, each domain gains control over its data, allowing for more precise and efficient data handling. This approach ensures the needs and expertise of each domain are directly aligned with how their data is managed, enhancing both the relevance and utility of the data.
2. Data as a Product
In Data Mesh, data is conceptualized not just as an asset, but as a product. This means that each set of data, or 'data product,' is treated as if it has its own lifecycle, including phases such as development, maintenance, and retirement.
Developing a data product involves understanding its specific use cases, target users, and the quality standards it must meet. It requires a clear definition of the product’s purpose, ensuring it aligns with user needs, and maintaining high standards of data quality and reliability. This approach to data management shifts the focus from mere data storage and processing to creating value-driven, user-centric data products.
3. Self-Serve Data Infrastructure
Self-serve data infrastructure is a pivotal principle in Data Mesh. It empowers individual domains within an organization to manage their data independently, without the need for constant intervention or support from central data teams.
Domains are provided with tools and resources to access, use, and manage their data autonomously. This infrastructure fosters agility and responsiveness, allowing domains to quickly adapt to changing needs and opportunities. It accelerates innovation and reduces bottlenecks in data access and utilization, as each domain can directly address its data requirements.
4. Centralized Governance Standards
In the context of Data Mesh, while data ownership and management are decentralized, governance standards are centralized. This means that although individual domains within an organization have control over their own data, there is a set of overarching governance standards that apply across all domains.
Centralized governance standards in a Data Mesh environment play a critical role in ensuring that, while domains are empowered to manage their data independently, they do so in a way that aligns with the overall objectives, security protocols, and compliance requirements of the organization.
Data Mesh vs Traditional Data Management
To fully appreciate the advantages of a Data Mesh approach, it’s important to understand the key differences between Data Mesh and traditional centralized data management models:
Centralized Data Management: The Traditional Model
Centralized data management typically involves a central data team responsible for the collection, storage, and processing of data. This data is often housed in a centralized repository, such as a data warehouse or lake. The primary characteristic of this model is that it centralizes data control and governance, often leading to a few key challenges:
-
Delays in Data Access and Processing: With a centralized system, there can be significant delays in accessing and processing data due to the bottleneck created by the central data team. This team is responsible for handling requests from various departments, which can lead to a backlog and slower response times.
-
Inefficiencies: Centralized systems often struggle with inefficiencies. Since the central team might not have specialized knowledge in every domain, they may not be able to optimize the data for specific uses, leading to suboptimal data utilization.
-
Disconnect Between Data Movers and Data Users: In this model, those who create, gather, and move data (movers) are often separated from those who use the data (users). This separation can lead to misunderstandings about the data's context, relevance, and potential applications.
Data Mesh: The Distributed Approach
Data Mesh represents a shift towards a distributed model of data management. In this approach, data is managed as a product by domain experts within different segments of an organization. This model addresses many of the challenges of centralized systems:
-
Faster Decision-Making: Since data is managed by domain experts who are closer to its context and application, decisions can be made more quickly. These experts have a better understanding of the specific needs and can tailor data management processes to suit these requirements.
-
Improved Data Quality: Data quality is often higher in a Data Mesh model. Domain experts are more likely to understand and maintain the integrity and relevance of the data, as they are directly involved in its creation and use.
-
More Efficient Data Operations: Data operations become more efficient under Data Mesh. Since data handling is decentralized, it reduces the burden on a central team and allows for quicker modifications and improvements in data management practices.
Key Differences and Benefits
-
Speed and Agility: Data Mesh promotes quicker access to data and faster decision-making due to the proximity of data management to its use.
-
Data Quality and Relevance: With domain experts handling the data, there is a higher likelihood of maintaining its quality and relevance for specific applications.
-
Operational Efficiency: Decentralizing data management leads to more efficient operations.
Data Mesh vs Data Fabric
In the realm of modern data architecture, Data Mesh and Data Fabric are often discussed, but it’s crucial to understand that they are not opposing strategies but rather complementary approaches, each focusing on different aspects of data management.
Data Mesh: An Organizational Design Approach
Data Mesh is primarily an organizational design approach. It focuses on how data is managed within an organization, emphasizing the decentralization of data ownership and management. In Data Mesh, data is treated as a product, with each business domain or team taking responsibility for their data. This approach promotes domain expertise in data management and aligns data closely with the specific needs and context of each domain.
-
Core Principle: Decentralization and domain-oriented data ownership.
-
Focus: Organizational structure and process around data management.
-
Benefit: Enhances data quality and accelerates decision-making within domains.
Data Fabric: Focused on Infrastructure
Data Fabric, in contrast, is more about the infrastructure for data management. It aims to create a unified metadata framework across the organization, integrating data from various sources into a single, accessible, and coherent structure. Data Fabric leverages technologies like AI and ML for automation in data discovery, governance, and integration, ensuring seamless access to data across the organization.
-
Core Principle: Unified data infrastructure and integration.
-
Focus: Technological solutions for data integration and accessibility.
-
Benefit: Provides a comprehensive view of all organizational data, making it easily accessible and manageable.
Complementary Nature
-
Infrastructure and Organization Alignment: Data Fabric can provide the technological infrastructure necessary for a Data Mesh implementation. The unified data layer of a Data Fabric can facilitate the distribution and accessibility of data across different domains in a Data Mesh architecture.
-
Enhanced Data Management: While Data Mesh addresses the organizational and process aspects of data management, Data Fabric provides the technological underpinning. Together, they can create a more robust, efficient, and effective data management strategy.
-
Broad Scope and Domain-Specific Focus: Data Fabric’s broad scope of integrating data across the organization complements Data Mesh’s focus on domain-specific data management. This combination allows organizations to have both a macro and micro perspective on their data.
Data Mesh and Data Fabric should not be viewed as mutually exclusive or competing concepts, but rather as complementary. Data Mesh’s organizational design approach, combined with Data Fabric’s infrastructure capabilities, can provide a comprehensive and efficient framework for managing an organization’s data landscape.
Data Mesh Use Cases and Impact
From finance to education, Data Mesh is redefining how organizations manage and utilize data, driving significant improvements in their processes and outcomes:
Finance: Risk Management and Customer Insights
In finance, Data Mesh is revolutionizing risk management and customer insights. Banks and financial institutions now use domain-specific data for rapid risk assessment and personalized customer service. This leads to improved decision-making and customer satisfaction. For example, credit risk assessment has become more accurate, reducing default rates, while personalized investment advice improves customer relations.
Manufacturing: Supply Chain and Production Efficiency
Manufacturers are using Data Mesh to optimize supply chains and enhance production efficiency. This approach provides a comprehensive view of operations, allowing for proactive responses to disruptions. The result is increased efficiency, reduced costs, and improved productivity, directly benefiting profitability and market competitiveness.
Retail: Customer Analysis and Inventory Management
In retail, Data Mesh transforms customer behavior analysis and inventory management. Stores and online channels use their data to customize experiences and manage stock levels efficiently. This leads to personalized product recommendations, better inventory control, and an enhanced shopping experience for customers.
Healthcare: Patient Data and Research
Healthcare institutions are advancing patient data management and research with Data Mesh. Departments like oncology and cardiology independently manage their data, improving patient care and speeding up research. This has led to more personalized treatments, better patient monitoring, and more efficient healthcare coordination.
Government: Public Services and Policymaking
Government agencies use Data Mesh to optimize public services and policymaking. Data from departments like transportation and health is managed independently, enhancing policy development and service delivery. For instance, improved data on traffic patterns can lead to better transportation planning, benefiting citizens' daily lives.
Education: Student Data and Institutional Planning
Educational institutions leverage Data Mesh for better student data analysis and institutional planning. Faculties and departments manage their data independently, enhancing educational quality and operational efficiency. This results in tailored educational support for students and data-driven institutional strategies, improving the overall educational experience.
Implementing Data Mesh
Implementing Data Mesh in an organization is a transformative process that involves several key steps, from the initial assessment to the full-scale execution. Here's how to approach it:
Step 1. Initial Assessment and Planning
The first step in implementing Data Mesh is to assess the current data landscape. This involves understanding the existing data practices, infrastructure, and capabilities of the organization. During this phase, it's important to identify the different domains within the organization that will take ownership of their data under the Data Mesh model.
Aligning stakeholders with the Data Mesh philosophy is also crucial at this stage. This means educating them about the benefits of decentralized data management and how it differs from traditional approaches.
Step 2. Defining Domains and Responsibilities
Once the assessment is complete, the next step is to clearly define each domain and its associated responsibilities. Domains are typically defined based on business functions or areas of expertise, such as marketing, sales, operations, etc. In this phase, it's important to assign data ownership to the appropriate domain teams.
These teams will be responsible for the creation, maintenance, and quality of their data. Clear communication and documentation are key to ensuring that each domain understands its role and responsibilities in the Data Mesh framework.
Step 3. Setting Up Infrastructure and Tools
Implementing Data Mesh requires a robust technological infrastructure. This includes setting up storage platforms that support decentralized data management, integration tools to facilitate data sharing and collaboration between domains, and data governance systems to maintain data quality and compliance.
The infrastructure should be flexible enough to accommodate the specific needs of each domain while ensuring overall cohesion and compatibility within the organization.
Step 4. Training and Culture Change
A crucial aspect of implementing Data Mesh is the training and culture change required for domain teams. Since Data Mesh represents a significant shift from traditional data management practices, comprehensive training is essential to ensure that domain teams are equipped to manage their data effectively.
Additionally, promoting a culture change towards a more collaborative, domain-driven approach is critical. This involves fostering a sense of ownership and accountability among domain teams for their data products.
Step 5. Iterative Implementation and Scaling
Implementing Data Mesh should be an iterative process. Start with a pilot domain to test and refine the implementation process. This pilot phase allows the organization to identify challenges and gather feedback, which can be used to improve the process for subsequent domains.
Once the pilot is successful, the Data Mesh can be scaled progressively to other domains. This gradual scaling allows for continuous learning and adaptation, ensuring that the implementation is effective and sustainable.
Implementing Data Mesh requires careful planning, clear definition of roles and responsibilities, robust infrastructure, comprehensive training, and an iterative approach. Each step is crucial for ensuring that the transition to a decentralized data management model is successful and brings about the intended benefits of agility, efficiency, and improved data quality.
Best Practices and Strategies for Implementation
Implementing Data Mesh effectively requires robust data governance strategies. This section covers best practices for ensuring data quality, privacy, and compliance within a decentralized data architecture:
Effective Data Governance in Data Mesh
Effective data governance is a cornerstone of successful Data Mesh implementation, ensuring compliance and strategic alignment across all domains:
-
Privacy and Compliance Frameworks: Develop comprehensive privacy policies and compliance frameworks that align with legal requirements like GDPR or HIPAA, taking into account the specific data types and uses within each domain. Ensure that these frameworks are adaptable to the diverse data landscape of a Data Mesh. Regularly assess and update privacy and compliance measures to stay current with evolving regulations and emerging risks.
-
Centralize Governance Standards: Adopt a federated governance model that strikes a balance between domain autonomy and centralized oversight. Empower individual domains to manage their data while ensuring adherence to organization-wide data policies and standards. Establish clear roles and responsibilities for data stewards within each domain, responsible for data quality and compliance, and create channels for communication and collaboration between domains to address common challenges.
-
Regular Training and Awareness Programs: Conduct ongoing training sessions to keep domain teams updated on governance policies, privacy norms, and compliance requirements. These training programs should be tailored to the specific needs of each domain and include practical examples and case studies. Additionally, foster awareness of the importance of data governance throughout the organization by regularly communicating success stories and the tangible benefits it brings.
Promoting a Data-Empowered Culture
Creating a culture where data is democratically accessible and collaboratively used is essential for maximizing the benefits of Data Mesh. This requires a comprehensive approach to education and change management:
1. Fostering Cross-Domain Collaboration: Encourage cooperation and knowledge sharing between different domains. Facilitate regular cross-domain meetings and workshops where teams can share insights, challenges, and best practices. By fostering an environment of collaboration, domains can leverage each other's expertise and drive innovation through collective intelligence.
2. Building Data Literacy: Invest in building data literacy across the organization. Provide comprehensive training and resources to help employees understand and leverage data in their respective roles. Offer a range of learning opportunities, from basic data literacy courses to advanced data analysis and visualization training. Promote a culture where employees feel confident in their ability to interpret and use data effectively.
3. Empowering Teams with Self-Service Tools: Implement self-service data tools that allow users, including non-technical domain experts, to access and analyze data independently. Empowering teams with user-friendly, self-service analytics platforms reduces dependency on IT or data specialists, enabling faster and more agile decision-making processes. Ensure these tools are user-centric, with intuitive interfaces and comprehensive training and support.
4. Celebrating Data-Empowered Successes: Recognize and celebrate instances where data-empowered approaches have led to significant achievements or innovations within the organization. Share success stories and case studies that highlight the value of data-empowered decision-making. These celebrations not only motivate and inspire teams but also reinforce the importance of a data-empowered culture throughout the organization. Recognize and reward individuals or teams that contribute to data-driven successes, further encouraging a culture of data excellence.
By implementing these best practices and strategies, organizations can successfully navigate the complexities of Data Mesh, ensuring effective data governance and cultivating a data-empowered culture that maximizes the potential of decentralized data ecosystems.
Addressing Obstacles in Adopting a Data Mesh Approach
Implementing a Data Mesh approach in an organization can encounter several obstacles. Addressing these effectively is key to a successful transition. Here are some common challenges and strategies to overcome them:
1. Cultural and Organizational Resistance
One of the primary challenges in adopting Data Mesh is the cultural and organizational resistance to change. Traditional data management practices are deeply ingrained in many organizations, and shifting to a decentralized approach can be met with skepticism.
Strategy for Tackling Resistance: To address this, it's important to demonstrate the tangible value of decentralized data management. This can be done through case studies, pilot projects, and clear communication of the benefits, such as increased agility, better data quality, and faster decision-making. Fostering a culture of collaboration and shared responsibility is also crucial. Encouraging open communication, transparency, and cross-functional teamwork can help ease the transition and build support for the new approach.
2. Complexity in Integration and Interoperability
Another significant challenge is managing the complexity associated with integrating and ensuring interoperability between different data domains.
Managing Complexity: To manage this complexity, robust integration tools are essential. These tools should facilitate seamless data sharing and collaboration across different domains. Additionally, establishing clear standards for data interoperability is crucial. These standards should address data formats, protocols, and communication methods to ensure that despite the decentralized nature of data management, there is a cohesive and unified approach to how data is handled and shared across the organization.
3. Ensuring Data Quality
Maintaining high standards of data quality in a decentralized environment like Data Mesh can also be challenging.
-
Data Quality Standards: Define clear data quality standards and guidelines that apply uniformly across all data domains. These standards should cover data accuracy, completeness, consistency, timeliness, and relevancy. Ensure that these standards are well-documented and readily accessible to all teams.
-
Data Profiling and Monitoring: Implement data profiling and monitoring tools that continuously assess the quality of data across different domains. Automated data quality checks can help identify and flag issues in real-time. Establish metrics and rules to measure data quality and set up alerts for deviations from the defined standards.
-
Data Quality Ownership: Assign data quality ownership responsibilities within each data domain. Each team or domain should have individuals or roles responsible for monitoring and improving data quality. Encourage a culture of accountability where data quality is everyone's responsibility.
Addressing the obstacles in adopting a Data Mesh approach requires a multifaceted strategy. By addressing these challenges head-on, organizations can effectively transition to a Data Mesh approach and reap its benefits.
Tools and Technologies for Data Mesh Implementation
Implementing a Data Mesh architecture requires a range of tools and technologies that support the decentralized management of data. These tools are essential for facilitating the various aspects of Data Mesh, from data integration to governance and collaboration.
Here's an overview of the key types of tools that are instrumental in implementing Data Mesh:
1. Data Integration and Management
Data integration and management tools are at the heart of a Data Mesh implementation. These tools enable the integration, processing, and management of data across multiple domains within an organization.
-
Functionality: These tools provide functionality for data ingestion, preparation, and delivery, ensuring that data from various sources can be effectively integrated and made available for use across different domains.
-
Key Features: TimeXtender excels in this area by offering a highly efficient and user-friendly environment for data integration and management. It streamlines the data ingestion and transformation process, ensuring seamless integration of data from diverse sources. Additionally, TimeXtender's ability to automate many aspects of data integration reduces manual effort and increases accuracy, making it an ideal choice for organizations implementing a Data Mesh architecture.
2. Metadata Management Systems
Metadata management systems are crucial for maintaining a comprehensive metadata repository in a Data Mesh environment.
-
Role of Metadata: Metadata plays a key role in data discovery, governance, and cataloging. It provides critical information about data sources, structure, and lineage, which is essential for effective data management and use.
-
Benefits: A robust metadata management system aids in organizing and accessing data across the decentralized domains. It supports data governance by providing insights into data quality, usage, and compliance.
-
TimeXtender's Unified Metadata Framework: TimeXtender's approach to metadata management stands out with its unified metadata framework. This framework centralizes metadata storage and management, making it easier for organizations to access, understand, and govern their data across various domains. By providing a holistic view of metadata, TimeXtender enhances data discoverability and governance, ensuring that data assets are consistently managed and effectively utilized.
3. Data Governance and Quality Tools
To ensure that data within each domain adheres to quality standards and compliance requirements, data governance and quality tools are necessary.
-
Governance Tools: These tools help in establishing and enforcing data policies, standards, and practices across the organization. They are essential for managing data access, security, and compliance.
-
Quality Tools: Data quality tools are used to monitor, clean, and maintain the integrity of data. They help in identifying and correcting data issues, ensuring that data is accurate, consistent, and reliable.
-
Holistic Governance with TimeXtender: Timextender provides robust data governance tools that enable organizations to establish and enforce data policies, standards, and practices seamlessly. These tools play a crucial role in managing data access, ensuring data quality, and maintaining compliance, thus fostering a secure data mesh environment.
4. Observability and Monitoring Tools
Observability and Monitoring Tools are indispensable components in modern data ecosystems, offering organizations the ability to gain insights into the performance, reliability, and health of their systems.
-
Comprehensive Observability: Observability tools provide organizations with a holistic view of their data ecosystems. These tools enable real-time tracking and analysis of data flows, allowing for the identification of issues, bottlenecks, and anomalies. This comprehensive observability ensures that data remains reliable and accurate throughout its journey within the organization.
-
Data Lineage and Documentation: Data lineage tools and documentation practices are essential for tracking the origin, transformations, and dependencies of data within an organization's ecosystem. They offer transparency into how data moves through the system, helping organizations maintain data quality, compliance, and the ability to troubleshoot issues efficiently.
-
Holistic Data Observability with TimeXtender: Timextender goes beyond conventional data management solutions by offering a comprehensive suite of features for data observability, lineage, and documentation. With Timextender, organizations can gain real-time insights into data flows, track data lineage from source to destination, and automatically maintain detailed documentation of their data processes.
The successful implementation of Data Mesh requires a comprehensive set of tools and technologies. These tools collectively support the decentralized yet coordinated management of data, ensuring that the organization's data assets are maximized for value generation.
The Future of Data Mesh
As Data Mesh gains traction, it's being influenced by evolving technological trends and shifts in the data landscape. These developments are not only enhancing its capabilities but are also shaping its future trajectory. Here’s a look at the emerging trends and their implications for Data Mesh:
Emerging Trends in Data Mesh
-
Integration with AI Technologies: Data Mesh is increasingly integrating with cutting-edge technologies such as artificial intelligence (AI) and machine learning (ML). This integration is poised to enhance the capabilities of Data Mesh, enabling sophisticated data processing, analytics, and automated decision-making. This trend suggests a future where Data Mesh systems not only manage and distribute data but also actively contribute to generating insights.
-
IoT and Edge Technology: The integration of Internet of Things (IoT) and edge technology is a significant emerging trend in Data Mesh. As IoT devices become more prevalent, generating vast amounts of data at the network's edge, Data Mesh architectures are adapting to leverage this data effectively. By integrating with edge computing technologies, Data Mesh can process and analyze data closer to its source, leading to faster insights and responses. It underscores the evolving need for Data Mesh to handle not just the volume and variety of data, but also the velocity and location, making data management more responsive and efficient in an increasingly connected world.
-
Focus on Data Privacy and Security: With increasing global emphasis on data privacy and security, Data Mesh is evolving to incorporate more robust privacy controls and security measures. This trend is in response to regulatory requirements like GDPR and the growing awareness of data sovereignty issues. Data Mesh models are being designed to ensure that data privacy and security are integral, especially when managing sensitive or personal data.
-
Greater Emphasis on Data Governance: As Data Mesh models become more widespread, there’s a growing emphasis on establishing robust data governance frameworks. These frameworks are essential for ensuring data quality, compliance, and effective management across decentralized domains. This trend reflects the need for a balance between the autonomy of domain-specific data management and the overarching governance standards of the organization.
Predictions and Outlook for Data Mesh
Looking into the future, Data Mesh is set to reshape data strategies in several key ways:
-
Decentralization as Standard Practice: The decentralized approach championed by Data Mesh is expected to challenge and possibly become the norm over traditional centralized data management models. This shift would represent a significant change in how organizations handle and perceive data.
-
Widespread Industry Adoption: As the benefits of Data Mesh become more apparent, its adoption is expected to extend across various industries. Each sector, with its distinct data challenges and needs, will find value in the flexibility and efficiency that Data Mesh offers.
-
Cultivating a Data-Empowered Culture: Data Mesh facilitates the democratization of data access and management within organizations. This approach is likely to continue driving a more data-empowered culture, where data becomes a central pillar in decision-making processes across all levels of the organization.
-
Boosting Organizational Agility: Data Mesh's ability to provide quick access to relevant and high-quality data will likely be instrumental in enhancing organizational agility. This will enable businesses to respond more swiftly and effectively to market changes and emerging opportunities.
As it continues to develop, Data Mesh is poised to become a cornerstone in modern data management strategies, heralding a new era of decentralized, efficient, and responsible data handling.
Empowering a Decentralized Approach with TimeXtender
TimeXtender serves as a holistic solution that addresses both the foundational aspects of data infrastructure and the tooling needs of data product creation. Its dual-purpose functionality ensures that your organization can seamlessly tackle infrastructure and governance concerns while also meeting the decentralized tooling needs essential for implementing a robust data mesh strategy:
Data Fabric Builder for Centralized Infrastructure and Governance: TimeXtender's Data Fabric Builder is the backbone of your data infrastructure and governance strategy. It provides a centralized platform that seamlessly integrates data from diverse sources, ensuring a unified view of your organizational data. With robust data governance capabilities, it allows you to implement data policies, track data lineage, and enforce security protocols, safeguarding data integrity and compliance with industry regulations.
Data Product Builder for Decentralized Data Product Development: Simultaneously, TimeXtender’s Data Product Builder is uniquely equipped to cater to the decentralized tooling requirements inherent in a data mesh approach. It offers an intuitive, drag-and-drop interface that enables business intelligence experts, analysts, and domain experts to collaboratively build and maintain data products without the need for extensive coding or specialized technical skills. This democratization of data product creation fosters agility within your organization, as teams can independently develop and deploy data products, reducing bottlenecks and accelerating time-to-insights.
.png?width=3561&height=2740&name=Data%20Product%20Builder%20(5).png)
TimeXtender, with its holistic suite of features and capabilities, significantly enhances the implementation of Data Mesh in organizations. It aligns with the key components of Data Mesh, providing benefit-oriented solutions that cater to the needs of a decentralized data environment:
Decentralized Data Ownership
-
Empowering Domains with Autonomy: TimeXtender supports decentralized data ownership by empowering individual domains to manage their data autonomously. Its user-friendly interface allows domain experts to handle data product creation and management without relying heavily on IT resources. This autonomy fosters a sense of ownership and encourages domains to align data management with their specific needs and expertise.
Data as a Product
-
Lifecycle Management of Data Products: TimeXtender treats data as a product, offering functionalities that support the entire data lifecycle, from development to retirement. This holistic approach ensures that data products are consistently managed, maintained, and updated to meet evolving user needs and business objectives.
-
Quality and Reliability Assurance: TimeXtender emphasizes maintaining high standards of data quality and reliability. Its robust data quality tools ensure that data products are dependable and useful for decision-making, reinforcing the value-driven, user-centric nature of data in a Data Mesh framework.
Self-Serve Data Infrastructure
-
Enabling Efficient Data Access and Management: TimeXtender’s self-serve data infrastructure empowers users across domains to access, use, and manage data independently. This reduces bottlenecks in data access and utilization, accelerating innovation and decision-making processes within domains.
-
Resource Optimization: By providing a self-serve infrastructure, TimeXtender allows domains to optimize their resources, focusing on value-add activities rather than data management intricacies.
Centralized Governance Standards
-
Unified Governance Framework: While promoting decentralized data ownership, TimeXtender ensures adherence to centralized governance standards. It offers a unified framework for data governance, ensuring consistency, compliance, and security across all domains.
-
Balancing Autonomy with Control: TimeXtender strikes a balance between domain autonomy and organizational control, ensuring that while domains manage their data independently, they do so within an established framework of governance standards.
Integration and Scalability
-
Seamless Integration Across Diverse Data Sources: TimeXtender excels in integrating a wide range of data sources, crucial for organizations employing a Data Mesh architecture. It efficiently connects and harmonizes data from various domains, regardless of the original format or location. This capability ensures that data across the organization can be easily accessed and utilized, supporting the collaborative nature of Data Mesh.
-
Future-Proof Scalability to Match Organizational Growth: TimeXtender's architecture is designed to be both technology-agnostic and future-proof, ensuring that it can seamlessly scale to match an organization's evolving data needs. This scalability is essential in a Data Mesh environment, where data volume, variety, and velocity can grow rapidly. TimeXtender's platform adapts to these changes without being tied to specific technologies, thus providing a durable and flexible foundation that supports long-term growth and adaptation in an ever-evolving data landscape.
AI-Powered Automation
-
Enhancing Data Operations with AI: TimeXtender leverages AI-powered data automation to streamline various aspects of data management within the Data Mesh framework. This includes automating routine data tasks like ingestion, transformation, and quality checks. AI-driven automation not only saves time but also reduces the likelihood of human error, enhancing the overall quality and reliability of data.
.png?width=6432&height=4125&name=Data%20Product%20Builder%20(10).png)
In summary, TimeXtender's holistic capabilities bridge the gap between infrastructure and governance concerns, and the decentralized tooling needs of a successful data mesh strategy.
Try TimeXtender for Free!
Click here to get started with a FREE trial and try out all the capabilities you need to create a powerful data mesh and unlock the full potential of your data, without a large team or a complex stack of expensive tools!