5 min read
Rise of the Machines: The Impact of Artificial Intelligence and Machine Learning on Data Automation and Integration
Written by: Aaron Powers, Content Marketing Specialist - July 27, 2023
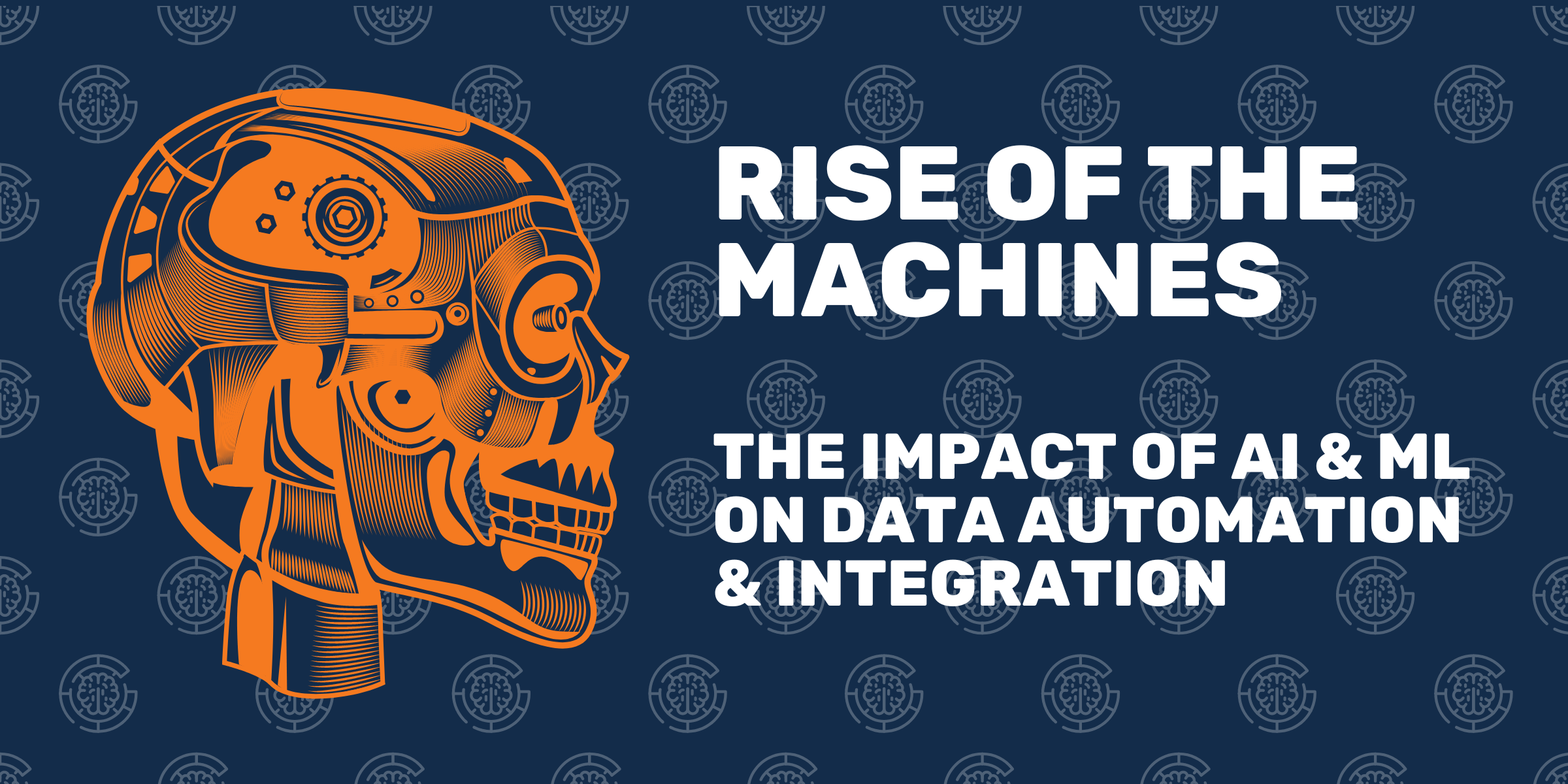
Ask anyone, and they’ll tell you their favorite Terminator movie is not Rise of the Machines (it’s T2: Judgment Day, in case you were wondering). While there have been numerous Terminator movies over the years, some good and some bad (we’re looking at you, Genisys), people have been fascinated with the idea of artificial intelligence (AI) and sentient machines long before James Cameron brought us the first Terminator in 1984.
AI has been a part of science fiction for decades, and as with any good science fiction, that science has become part of reality due to amazing technological advances and significant strides made in robotics, computer systems, and more. When it comes to data automation and integration, AI and machine learning (ML) have greatly impacted how data is stored, used, and transformed. At TimeXtender, we know that these advances will only continue to accelerate, changing how organizations look at and ultimately apply their data.
How Have AI and ML Impacted Data Automation and Integration?
With new ideas being thought of and implemented all the time, it’s nearly impossible to tell what impact AI and ML will have on data automation and integration in the future. What we do know is how they have changed the game thus far, including:
- Data Cleansing and Preprocessing: AI and ML algorithms can automatically clean and preprocess large datasets, identifying and rectifying errors, missing values, and inconsistencies. This streamlines the data integration process and ensures the data used for analysis is accurate and reliable.
- Data Mapping and Matching: AI-powered data integration tools can intelligently map and match data from different sources, even when the data is not uniform or structured similarly. This automation simplifies the integration of heterogeneous datasets, saving time and effort.
- Natural Language Processing (NLP) for Data Integration: NLP-based AI systems can interpret and extract relevant information from unstructured data sources such as text documents or emails. By understanding the context and meaning, they can integrate this unstructured data with structured datasets, enabling a more comprehensive analysis.
- Automated ETL (Extract, Transform, Load) Processes: AI and ML can automate the ETL process by learning from historical data transformation patterns. This reduces the need for manual intervention, allowing data to be integrated more quickly and efficiently.
- Predictive Data Integration: AI can predict data integration requirements based on historical patterns, user behavior, and other relevant factors. This helps proactively prepare for data integration needs, ensuring seamless and timely integration of new data.
- Anomaly Detection: AI-powered anomaly detection systems can automatically identify and handle inconsistencies or outliers in the data during integration. This ensures that any abnormal data points are appropriately addressed to prevent inaccurate analysis results.
- Smart Data Merging: AI algorithms can intelligently merge data from multiple sources, handling conflicts, duplicates, and overlapping records efficiently. This ensures a unified and coherent view of the data for analysis and decision-making.
- Data Integration Recommendations: AI can suggest optimal data integration strategies based on factors like data volume, source reliability, and the required analysis outcomes. This helps data analysts and engineers make informed decisions during the integration process.
- Intelligent Data Transformation: AI and ML can automate the data transformation process, adapting to changes in data sources and formats without human intervention. This flexibility allows for a more agile and adaptive data integration environment.
These examples demonstrate how AI and ML technologies have revolutionized data automation and integration, making it faster, more accurate, and efficient for businesses and organizations to harness the power of their data.
What Challenges Do AI and ML Present for Data Professionals?
As John Connor had to fight against Skynet, data pros across the globe have had their struggles with AI and ML. While meant to be user-friendly and convenient, AI and ML can present challenges that must be overcome in certain situations. Some of these challenges include:
- Expertise and Skillset: Implementing AI and ML in data automation can require specialized expertise and a deep understanding of these technologies. Data professionals may need to acquire new skills or collaborate with data scientists and AI specialists, which can be time-consuming and resource intensive.
- Data Quality and Governance: AI and ML models heavily rely on data quality for accurate results. Data professionals face the challenge of ensuring data integrity, consistency, and proper governance throughout the data automation and integration process.
- Integration Complexity: Integrating AI and ML models into an existing data infrastructure can be complex. Data teams need to ensure compatibility and seamless integration with various data sources and platforms.
- Security and Privacy: As AI and ML models handle sensitive data, data professionals must prioritize security measures to protect against data breaches and unauthorized access.
- Data Volume and Variety: The sheer volume and diversity of data generated in modern organizations pose challenges for data professionals when implementing AI and ML-based data automation. Handling big data efficiently requires robust infrastructure and optimization.
- Continuous Learning and Model Management: AI and ML models require continuous learning and updating to remain effective. Data professionals need to monitor model performance, adapt to changing data patterns, and manage model updates effectively.
- Interpreting Complex Models: Some AI and ML models are highly complex, making it challenging for data experts to interpret their decision-making processes and identify the factors driving specific outcomes.
- Resource Constraints: Implementing AI and ML in data automation may require significant computational resources, making it essential for data professionals to balance performance requirements with available infrastructure.
- Explainability and Transparency: Some AI and ML models operate as black boxes, making it difficult to explain their decisions. Data specialists may face challenges in ensuring model transparency and interpretability, especially in regulated industries.
- Adoption and Change Management: Integrating AI and ML into existing data workflows may encounter resistance from stakeholders who are unfamiliar with these technologies. Data pros need to address concerns and promote successful adoption within the organization.
Data professionals can overcome these challenges by investing in continuous learning, collaborating with experts from various fields, adhering to best practices, and adopting a data-centric approach to ensure successful AI and ML integration in data automation processes.
What Does the Future Hold?
As the Terminator movies have shown us, there are numerous possible futures based on the actions (and reactions) we take when it comes to AI and ML. At TimeXtender, we embrace the idea of faster, more efficient data automation and integration, especially when it helps organizations make better decisions with data that is actually reliable. Data observability, data quality, data lineage, and more can only benefit from advances in AI and ML, as they enable us to tackle complex data challenges with greater precision and scale. However, as we move forward, we must also be vigilant about the potential risks and challenges that AI and ML bring, such as data privacy concerns, biases in algorithms, and ensuring transparency and interpretability in AI-driven decision-making.
At TimeXtender, we’re committed to striking a balance between embracing the immense potential of AI and ML in data automation and integration while adhering to ethical principles and industry best practices. By prioritizing data governance and security, we aim to build trust and confidence in AI-driven processes, empowering organizations to leverage their data to its full potential. We’d be remiss if we didn’t mention our own AI data integration co-pilot, XPilot, which was designed to help users get the most out of our holistic data integration tool.
We believe that by embracing innovation while staying true to data integrity and transparency, we can pave the way for a future where data automation and integration are not only faster and more efficient, but also more reliable and ethically sound. Together, we can shape a future where AI and ML are powerful allies in unlocking the true value of data, driving meaningful insights, and shaping a more data-driven world for the betterment of all. No more worrying about Skynet becoming sentient and a T-800 smashing down our door and asking for our clothes, our boots, and our motorcycle.
Summary
AI and ML are integral parts of data automation and integration, just as they were for Cyberdyne Systems and the creation of Skynet. However, we can look forward to a bright future with AI and ML making automation and integration faster and easier than ever.
- AI and ML have impacted data automation and integration in the areas of data cleansing and preprocessing, data mapping and matching, NLP for data integration, automated ETL processes, predictive data integration, anomaly detection, smart data merging, data integration recommendations, and intelligent data transformation.
- Data professionals face challenges when implementing AI and ML, such as the need for specialized expertise, ensuring data quality and governance, integration complexity, security and privacy concerns, handling big data efficiently, continuous learning and model management, interpreting complex models, resource constraints, and promoting adoption and change management.
- It’s important to strike a balance between embracing AI and ML's potential and adhering to ethical principles, data governance, and transparency.
- TimeXtender is committed to ethical data practices and our AI data integration co-pilot, XPilot, is designed to assist data pros in achieving efficient data integration.
- The future of data automation and integration lies in leveraging AI and ML while prioritizing data integrity, transparency, and security, paving the way for a more data-driven and reliable world.
Just as John Connor says in T2, “The future’s not set. There’s no fate but what we make for ourselves,” the future of data automation and integration are what we make of it. Let's ensure we create a data-driven destiny with AI and ML as our trusted sidekicks, instead of rogue Terminators out to get us!