2 min read
Cloud Scale Analytics - TimeXtender & Microsoft Azure data platforms
Written by: TimeXtender - April 17, 2019
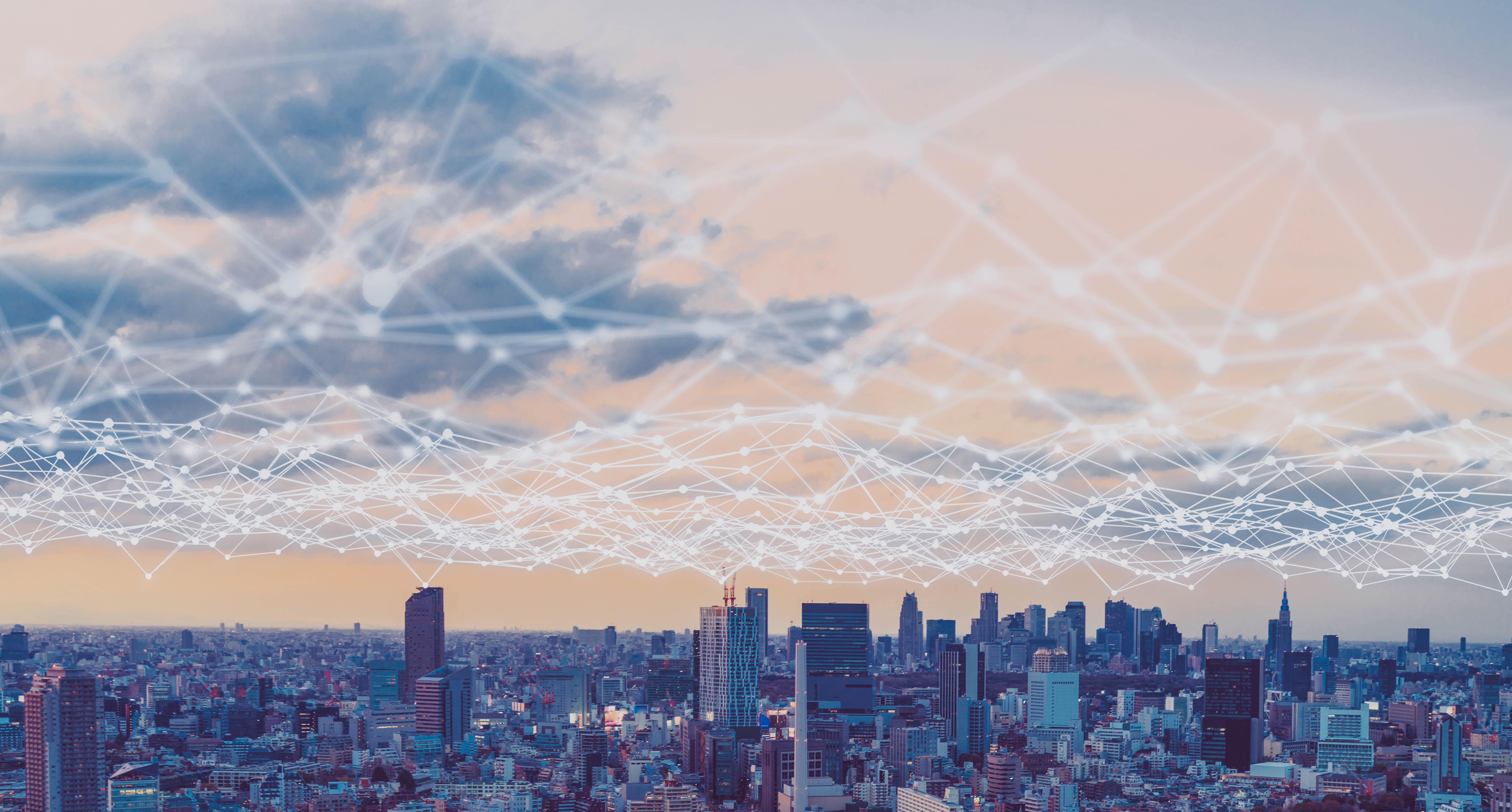
Exponential Data Growth
Data is constantly growing, we all know that… but it still surprised me to see some of the latest data predictions for 2020. For instance, did you know that by 2020, new information generated per second for every human being will amount to approximately 1.7 megabytes per second? Or that Google performs more than 40,000 searches per second and Facebook users send nearly 31 million messages every minute?
In the business and enterprise world, things are not much different. IDC estimates that by 2020, the number of business transactions (including both B2B and B2C) via the internet will reach 450 billion per day. While the volume of data created by U.S. companies alone each year will be enough to fill ten thousand Libraries of Congress (150 petabytes). What is more alarming is that only about 12% of this data is ever used or analyzed, leaving untold insights hidden and untouched in the other 88%.
Technology Changes
To keep up with this dramatic change, technology has been rapidly evolving as well. The cloud has become the de-facto standard for handling these dramatic data volumes with low cost data storage options like an Azure Data Lake proving to be one of the best and most popular ways of doing so. But just storing the data is not enough, we need to tap into insights hidden in the data - which means we need to process the data and make it available for business users to access it in a simple and easy to understand way.
Traditional methods for processing data through a relational database engine like SQL Server relied on a well-established process called Symmetric Multiprocessor (SMP). Simplistically, a SMP database assigns tasks/queries to a single CPU, regardless of how many CPU’s there are attached to the database. This type of processing just cannot keep up with the increased data processing demands. Massively Parallel Processor (MPP) architectures like Azure SQL Data Warehouse Gen2 have become the most effective way to process these large volumes of data. Queries on an MPP database are performed by each processor on the computers, in parallel, where segments of the database are stored and are processed significantly faster.
A simple example of how MPP is different from SMP, is to think of a physical warehouse. If you only had one loading dock, it would mean that you could only serve one truck at a time, forcing everyone else to que up. If you had 5 loading docks, you could easily serve 5 trucks at the same time and in doing so, reduce the wait time. In concept, performing tasks in parallel is the same way that MPP works to speed up the processing of data.
Cloud Scale Analytics
Combining these technologies (Azure Data Lake, Azure SQL Data Warehouse Gen2, etc.) into an end-to-end Cloud Scale Analytics architecture, is a proven method for gaining insight into enterprise data, but this requires a diverse set of skills including data engineers, data lake specialists, and data warehouse specialists.
TimeXtender is already a proven data management platform supporting many of the existing components of a full Cloud Scale Analytics architecture. In 2019, TimeXtender will add support for Azure SQL Data Warehouse Gen2, and in doing so, we will be able to cater to the end-to-end Cloud Scale Analytics reference architecture as below.
This means that customers will be able to take advantage of the unparalleled speed and ease of use of TimeXtender to build, deploy and manage both the architecture and the data pipelines, data models and semantic models.
Conclusion:
TimeXtender's proven capability in the data management space allows you to:
- seamlessly connect to data
- build, deploy, productionize and manage data engineering pipelines
- build, deploy, productionize and manage data models
- build, deploy, productionize and manage analytical/analysis models
- providing a comprehensive end-to-end platform to support compliance through data lineage and documentation of processes.
TimeXtender gives you the ability to build end-to-end data architectures and complete cloud scale analytic solutions that leverage the power of Azure SQL Data Warehouse Gen2’s parallel processing.