6 min read
Data Management Challenges for the Transportation & Logistics Industry
Written by: Micah Horner, Product Marketing Manager, TimeXtender - May 19, 2022
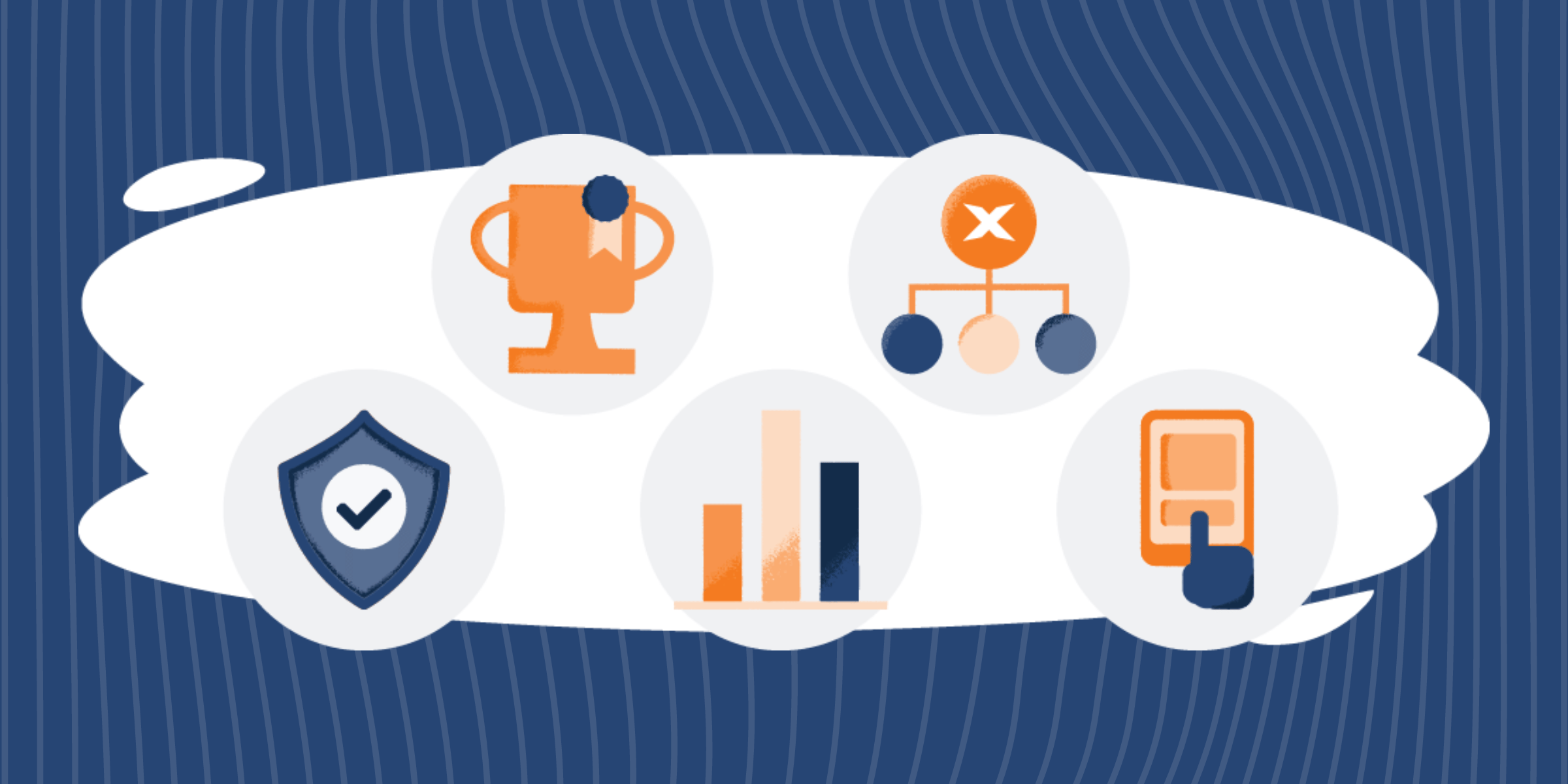
Supply chain management is at the forefront of everyone's minds these days, as shortages of goods and services continue to have ripple effects across the globe.
Logistics and transportation firms are still recovering from the aftermath of unprecedented pressures on global supply chains caused by the COVID-19 pandemic and subsequent lockdowns.
To add to these challenges, the industry is now contending with international conflicts, high fuel prices, surging ocean container rates, and the ever-present threat of driver shortages.
Amid all of this, successful firms are finding ways to leverage data and new technologies to cut costs, streamline operations, and improve customer service.
In fact, new era in the industry is emerging that will be powered by AI, machine learning, IoT, and other "smart" technologies in what's being referred to as the "Machine Economy."
It's not just hypothetical. These smart technologies are already permeating many aspects of the transportation and logistics sector.
Here are a few real-world examples:
- Predictive Analytics: Advanced data analytics and machine learning algorithms are being used to forecast traffic congestion, identify maintenance needs, and predict consumer demand.
- IoT Devices: A wide variety of IoT devices are being used in transportation and logistics, including GPS trackers, barcode scanners, and RFID tags. These devices are helping to improve asset tracking and management, as well as driver safety.
- Autonomous Machines: Autonomous vehicles, drones, and robots are increasingly being used for tasks such as package delivery, warehouse inventory management, and even long-haul trucking.
- Automated Customer Service: Automated customer support tools, such as chatbots and intelligent digital assistants, are enabling transportation and logistics firms to offer instant, personalized real-time support to their customers.
As the Machine Economy matures, transportation and logistics firms will continue to seek out new ways to use smart technologies to increase efficiency and improve customer service.
Unfortunately, the volume and variety of data being generated by these new "smart" technologies is stretching traditional data management infrastructures beyond their limits. Without an adequate solution, these data management challenges threaten to stifle innovation and put the future of the industry at risk.
Top 5 Data Management Challenges for the Transportation and Logistics Industry
1. Exploding Data Volumes
Data is the lifeblood of transportation and logistics companies. It is used to track shipments, plan routes, and manage inventory.
In the past, data was relatively static, with companies relying on manual processes to update and maintain their records. However, in recent years, data volumes have increased exponentially, as companies have started using sensors and other devices to generate real-time information about their operations.
This deluge of data has made it difficult for companies to keep up with the demand for information. As a result, many transportation and logistics companies are struggling to find ways to effectively manage the data.
2. Time-Consuming Data Preparation Tasks
Data scientists still report spending around 45% of their time just on data preparation tasks. Data preparation is an extremely tedious and time-consuming and costly process that often involves:
- Data discovery to identify data sources
- Data profiling to determine data attributes and data quality
- Data ingestion into data warehouses or data lakes using complex ETL processes
- Data cleansing to deal with data errors such as missing data, wrong data, or illogical data
- Data enrichment to fill in missing information such as location or date
- Data transformation to convert data from one format to another
Data preparation tasks are labor-intensive and error-prone, leading to issues with security and quality. Data accuracy can be compromised, data lineage is often unavailable, and data often does not reflect the latest state of the business.
/Textured%20Illustrations/cog-min.png?width=150&name=cog-min.png)
Increasing data volumes will only serve to exacerbate data preparation challenges, as data scientists will be forced to wade through more data than ever before just to extract the most useful data insights.
3. Talent Shortages
This assumes you already have a large data team of highly-specialized professionals who can do all this work. Demand for data science skills is outstripping supply, leaving many companies struggling to find enough talent.
Data engineering skills shortages are also starting to emerge. Data engineers are responsible for designing data pipelines and data architectures that can handle complex data management processes.
The lack of data engineers means that overworked data scientists end up spending more time on data engineering tasks than they would like, which slows down the rate at which they can extract valuable insights from their data.
The data science and engineering skills gap is widening due to several factors:
- Data skills are in high demand and data professionals can command increasingly high salaries
- Data science and data engineering are relatively new disciplines, so the data talent pool is still small
- Data scientists and data engineers require a diverse set of technical skills that aren't always easy to find in a single data professional
- The data preparation process itself is labor-intensive and requires a lot of patience and attention to detail, skills that can take years to develop
The talent shortage in the transportation and logistics industry is exacerbated even further by the fact that many of the most qualified data scientists and engineers are working in other industries, such as finance and healthcare.
4. Data Access and Security
Data security is a major concern for transportation and logistics companies, as they are often handling sensitive data such as customer data, employee data, and financial data. Data breaches can lead to loss of revenue, loss of customer trust, and legal penalties.
The challenge for transportation and logistics companies is to find the right balance between data security and data accessibility. Data needs to be secured against unauthorized access, but it also needs to be accessible to the people who need it to do their jobs.
These challenges are compounded by the fact that the data must be shared across a complex network of stakeholders, including government agencies, private companies, and international organizations. As a result, managing data in the transportation and logistics industry is a complex and demanding task.
Unfortunately, many organizations may not even know where all of their data is stored or if it's safe from unauthorized access. With so much data constantly changing—becoming more complex, diverse, and scattered across multiple devices—transportation and logistics firms need a data management strategy that is designed to keep data safe, accurate, and readily accessible.
5. Data Governance and Regulatory Compliance
Data governance is the process by which data is organized, managed, and controlled. This includes the identification, classification, and protection of data. The goal of data governance is to ensure that data is of high quality, and is used in a consistent and controlled manner, in compliance with all relevant laws and regulations.
The transportation and logistics industry is subject to strict safety regulations, which requires careful management of data related to accidents, incidents, and vehicle maintenance. Furthermore, the industry faces stringent environmental regulations, which requires detailed tracking of emissions data.
In addition to these specific regulatory requirements, transportation and logistics companies must also comply with general data privacy regulations, such as the EU's General Data Protection Regulation (GDPR).
Transportation and logistics companies that fail to comply with data privacy regulations can face heavy fines, and their reputation may be damaged.
With data volumes continuing to explode and the regulatory landscape becoming more complex, data teams will need to find data management tools that are not only scalable, but also flexible enough to adapt to data governance and compliance requirements.
Your Options: Stack, Platform, or Builder
Approach #1: The Stack
Traditionally, the data preparation process has relied on a highly-complex stack of tools, a growing list of data sources and systems, and months spent hand-coding each piece together to form fragile data “pipelines.”
Approach #2: The Platform
Then came data management “platforms” that promised to reduce complexity by combining everything into a single, unified, end-to-end solution. In reality, these platforms impose strict controls and lock you into a proprietary ecosystem that won’t allow you to truly own, store, or move your own data.
It’s clear that these old approaches to data management simply cannot meet the needs of data teams in the Machine Economy.
Data professionals are in desperate need of a faster, smarter, more flexible way to ingest and prepare their data for analysis, AI, and machine learning.
Fortunately, there is a third approach.
Approach #3: The Builder
In order to overcome the data management challenges listed above, data professionals need a solution that meets all three of these criteria:
- Low-Code: It must be smart enough to build your entire data estate for you by automatically generating all the underlying code and documentation, from end to end.
- Agile: It must provide both technical and business users with a simple, drag-and-drop user interface for quickly ingesting, preparing, and delivering corporate data for analytics and AI/machine learning.
- Integrated: It must seamlessly overlay your data storage infrastructure, with no vendor lock-in, while integrating all the data ingestion, preparation, quality, security, and governance capabilities you need into a simple, unified, metadata-driven solution.
Meet TimeXtender, the Low-Code Data Estate Builder
TimeXtender empowers you to build a modern data estate 10x faster by eliminating manual coding and complex tool stacks.
With our low-code data estate builder, you can quickly integrate your siloed data into a data lake, model your data warehouse, and define data marts for multiple BI tools & endpoints – all within a simple, drag-and-drop user interface.
TimeXtender seamlessly overlays your data storage infrastructure, connects to any data source, and integrates all the powerful data preparation capabilities you need into a single, unified solution.
Because all code and documentation are generated automatically, you can reduce build costs by 70%, free data teams from manual, repetitive tasks, and empower BI and analytics experts to easily create their own data products – no more bottlenecks.
We do this for one simple reason: Because Time Matters.
Learn How to Become Data Empowered with TimeXtender
Watch a demo to learn how we can help you build a modern data estate 10x faster, become data empowered, and win in the Machine Economy.